News
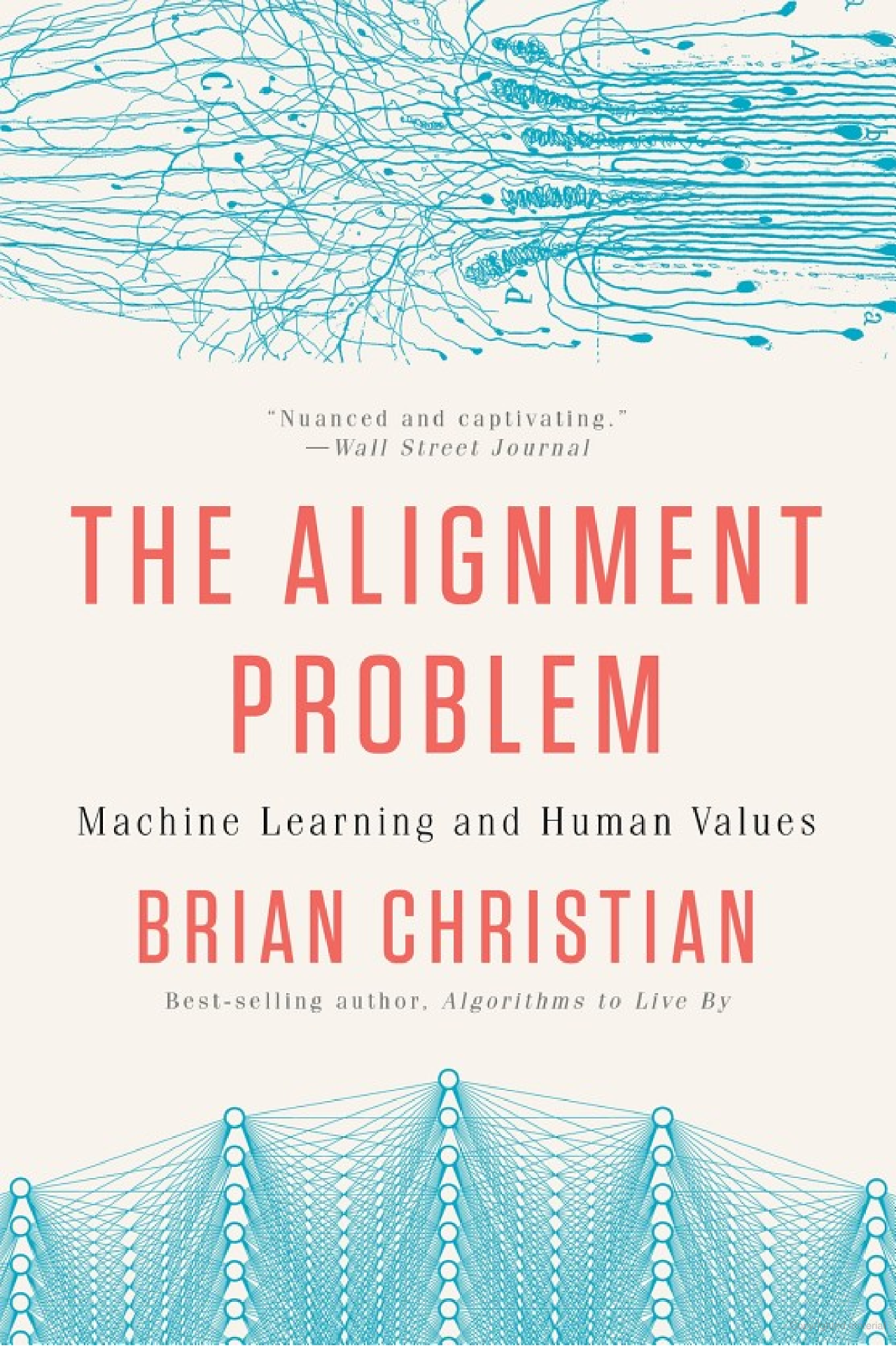
Brian Christian’s “The Alignment Problem” wins the Excellence in Science Communication Award From Eric & Wendy Schmidt and the National Academies
01 Nov 2022
Brian Christian has been named one of the inaugural recipients of the National Academies Eric and Wendy Schmidt Awards for Excellence in Science Communication
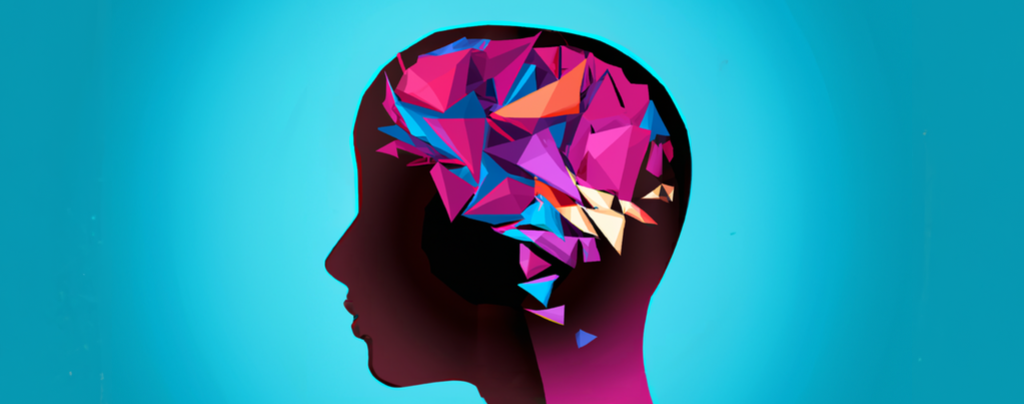
The Shard Theory of Human Values
14 Oct 2022
Starting from neuroscientifically grounded theories like predictive processing and reinforcement learning, Quintin Pope and Alex Turner (a postdoc at CHAI) set out a theory of what human values are and how they form within the human brain. In The shard theory of human values published in the AI Alignment Forum, they analyze the idea that human values are contextually activated influences on decision-making (“shards”) formed by reinforcement events. For example, a person’s reward center activates when they see their friend smile, which triggers credit assignment, which upweights and generalizes the person’s thoughts which led to the reward event (like “deciding to hang out with the friend” or “telling a joke”), creating a contextual influence (a “friendship-shard”) which, when the friend is nearby, influences the person to hang out with their friend again. This theory explains a range of human biases and “quirks” as consequences of shard dynamics.
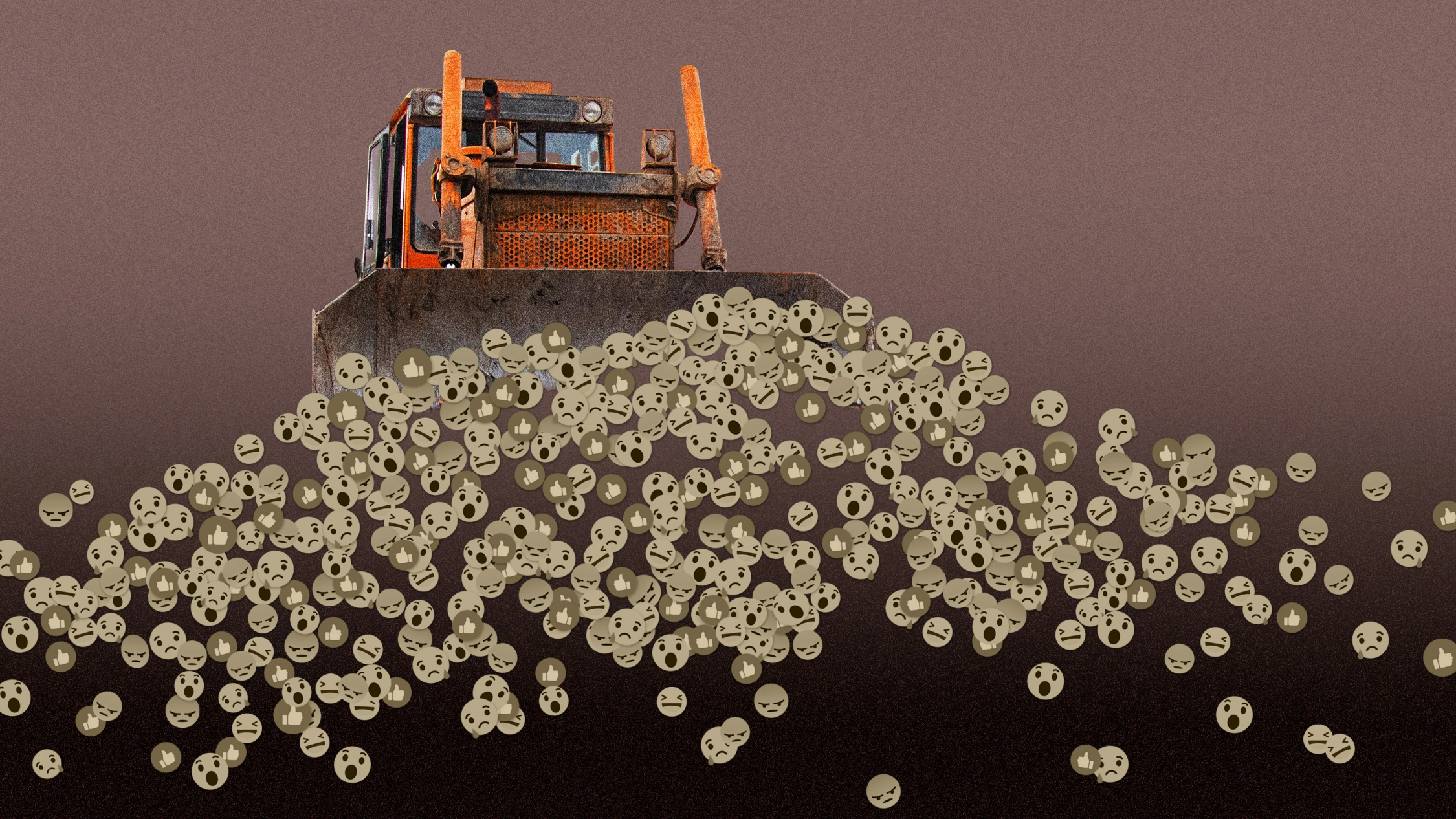
Social Media is Polluting Society. Content Moderation Alone Won’t Fix the Problem
10 Oct 2022
In Social media is polluting society. Content moderation alone won’t fix the problem published in the MIT Technology Review, CHAI’s Thomas Krendl Gilbert argues that if content moderation on social media were implemented perfectly, it would still miss a whole host of issues that are often portrayed as moderation problems but really are not. He explains that in order to address those non-speech issues, we need a new strategy: treat social media companies as potential polluters of the social fabric, and directly measure and mitigate the effects their choices have on human populations. That means establishing a policy framework—perhaps through something akin to an Environmental Protection Agency or Food and Drug Administration for social media—that can be used to identify and evaluate the societal harms generated by these platforms. If those harms persist, that group could be endowed with the ability to enforce those policies. But to transcend the limitations of content moderation, such regulation would have to be motivated by clear evidence and be able to have a demonstrable impact on the problems it purports to solve.
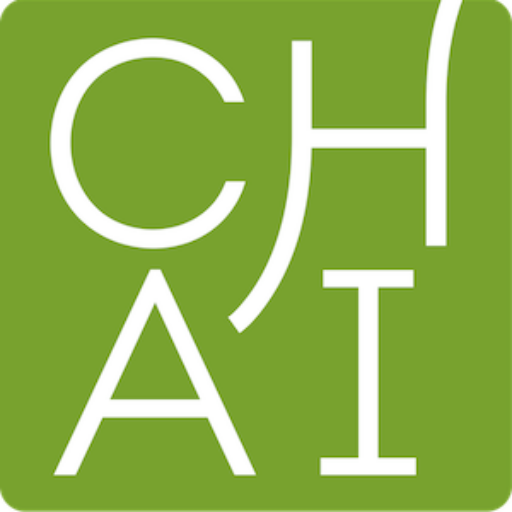
Relational Abstractions for Generalized Reinforcement Learning on Symbolic Problems
03 Oct 2022
In Relational Abstractions for Generalized Reinforcement Learning on Symbolic Problems, CHAI’s Siddharth Srivastava argues that reinforcement learning in problems with symbolic state spaces is challenging due to the need for reasoning over long horizons. This paper presents a new approach that utilizes relational abstractions in conjunction with deep learning to learn a generalizable Q-function for such problems. The learned Q-function can be efficiently transferred to related problems that have different object names and object quantities, and thus, entirely different state spaces. We show that the learned, generalized Q- function can be utilized for zero-shot transfer to re- lated problems without an explicit, hand-coded curriculum. Empirical evaluations on a range of problems show that our method facilitates efficient zero-shot transfer of learned knowledge to much larger problem instances containing many objects.