Spotlights
CHAI hosts weekly research meetings for graduate students at UC Berkeley. Below are samples of some of their work.
Dylan Hadfield-Menell
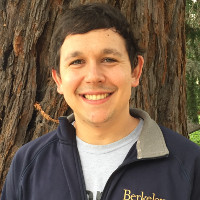
Dylan is a fourth year Ph.D. student at UC Berkeley advised by Anca Dragan, Pieter Abbeel, and Stuart Russell. His research focuses on algorithms that facilitate human-compatible artificial intelligence. In particular, he tries to develop frameworks that account for uncertainty about the objective being optimized.
Before coming to Berkeley, Dylan did a Master’s of Engineering with Leslie Kaelbling and Tomás Lozano-Pérez at MIT. At Berkeley, Dylan’s research has taken a turn to focus more on AI safety and, thinking longer term, AI value alignment:
- In 2016, he and his advisors formally described a cooperative inverse reinforcement learning problem (paper). The problem serves as a tool to help researchers consider how robots could learn humans’ values via cooperative instruction. While robots could learn humans’ values by observing humans, cooperative instruction is likely to be significantly faster.
- In 2017, Dylan and his advisors described an “off-switch game,” a simplified problem describing scenarios in which a human would like to turn off a robot, but the robot is able to disable its off-switch (paper). They showed that a robot who is uncertain about the utility derived from various outcomes in the game is more likely to allow a human to turn it off.
You can earn more about Dylan’s other professional work at his website and follow him on Twitter here.
Smitha Milli
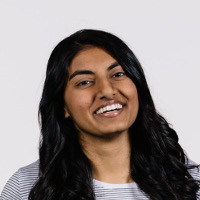
Smitha Milli is a first-year Ph.D. student at UC Berkeley, where she recently completed her undergraduate degree in EECS. She spent the summer of 2017 interning with OpenAI. Smitha wants to help create intelligent, collaborative AI systems that will have a positive impact on society.
During her undergraduate studies, Smitha worked with Stuart Russell (CHAI’s founder), Anca Dragan (a Principal Investigator at CHAI), and Dylan Hadfield-Menell (a CHAI graduate student) on the problem of how to make robots obedient, but not too obedient (paper). Because humans are fallible and sometimes give instructions that run counter to their preferences, it’s important to create robots that do not automatically obey all commands, but rather try to infer what a human desires. Smitha and her colleagues attempted to formalize this problem and assessed the extent to which various methods of inferring human preferences err on the side of obedience.
Going forward, Smitha wants to work on creating machine learning systems that are more robust and reliable. She is particularly interested in how interaction with humans can help ensure that systems have learned the right objectives and behavior.
You can learn more about Smitha and her work at her website and follow her on Twitter here.
Daniel Filan
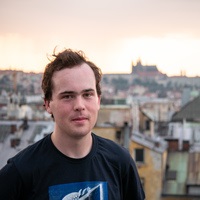
Daniel Filan is a 2nd-year Ph.D. student of EECS at UC Berkeley, supervised by Stuart Russell. He’s interested in effective altruism and wants to ensure that future artificial intelligences who may be much more strategically intelligent than us behave in a safe way.
In 2016, Daniel worked with Tom Everitt, Mayank Daswani, and Marcus Hutter, considering the problem that a sufficiently advanced AI could choose to modify its source code in order to have easily achievable goals, and such modifications may not be to humans’ liking (paper). They determined that an agent will not self-modify if and only if the value function of the agent anticipates the consequences of self-modification and uses the agent’s current utility function when evaluating the future.
Daniel is currently exploring how the task “create an AI whose interests are aligned with its user” is similar to the task of “write a contract to pay somebody for doing work for you.”, i.e. the Principal Agent problem in economics. He suspects there is insight to be gained from exploring the types of problems shared between both tasks, and how solutions to a problem for one of those tasks may be transferred to a similar problem for the other.
You can learn more about Daniel’s work at his personal website.
Vael Gates
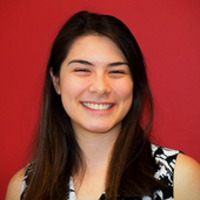
Vael Gates is a second-year Ph.D. student of Neuroscience at UC Berkeley, advised by Professor Tom Griffiths (a PI at CHAI). Her research is broadly aimed at developing computational models of social cognition. She is interested in:
- How people can infer beliefs and intentions in others, especially by observing others’ actions and employing recursive theory-of-mind (e.g. inverse reinforcement learning, social psychology);
- Group-level equilibria when agents are collaborating or competing (e.g. game theory, agent-based modeling);
- Mechanism design and other ways quantitative characterizations of a phenomenon can be used to predict and shape behavior.
Recently, Vael has worked on two projects related to CHAI’s mission. She has assisted with the paper on solving the cooperative inverse reinforcement learning (CIRL) dynamic game described in more detail in Jaime Fernandez Fisac’s profile and is working with Professors Anca D. Dragan, Tom L. Griffiths, and Anant Sahai on preference aggregation across agents. More specifically, in the latter project Vael and her colleagues have set up a study in which participants are presented with a problem that requires mediating between the preferences of multiple agents. Vael and her colleagues take participants’ responses and attempt to explain them using a quantitative model. Their hope is to create a baseline standard of “fair” reactions to the problem—a standard to which the behavior of future AIs can be compared.
Going forward, Vael would like research social inference problems. She wants to continue to approach social cognition from a computational perspective, using probabilistic models and large-scale web-based crowdsourcing to investigate the computational goals and algorithms driving the social mind. By understanding the complex inferences made by human minds, she hopes to contribute to the development of artificial intelligence that can collaborate and is compatible with human behavior.
You can learn more about Vael at her website.