News
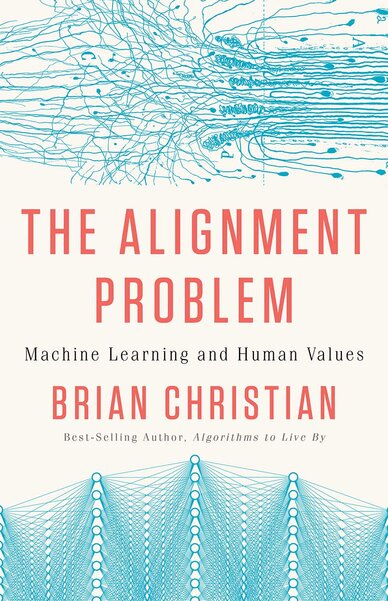
“The Alignment Problem” Wins Xingdu Book Award
29 Aug 2024
Brian Christian’s book “The Alignment Problem” was announced as the sole winner in the New Knowledge Category for Imported Editions at the Xingdu Book Award ceremony in China. The Chinese translation was published this past year by Hunan Science & Technology Press.
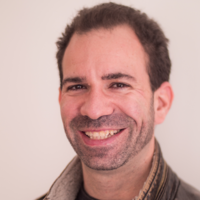
Forget deepfake videos. Text and voice are this election’s true AI threat.
08 Jul 2024
Jonathan Stray, Senior Scientist at CHAI, and Jessica Alter, tech entrepreneur and co-founder of Tech for Campaigns, wrote an op-ed for The Hill regarding the risks posed by AI in this current election cycle.
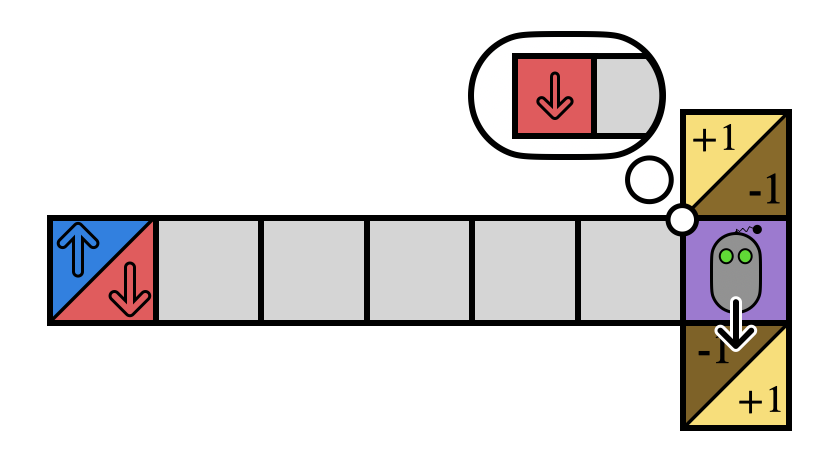
Mitigating Partial Observability in Decision Processes via the Lambda Discrepancy
28 Jun 2024
This paper investigates fundamental concepts related to detecting and mitigating partial observability by measuring misalignment between value function estimates. The paper was presented at the “Finding the Frame” workshop at RLC 2024 and the “Foundations of Reinforcement Learning and Control” workshop at ICML 2024.
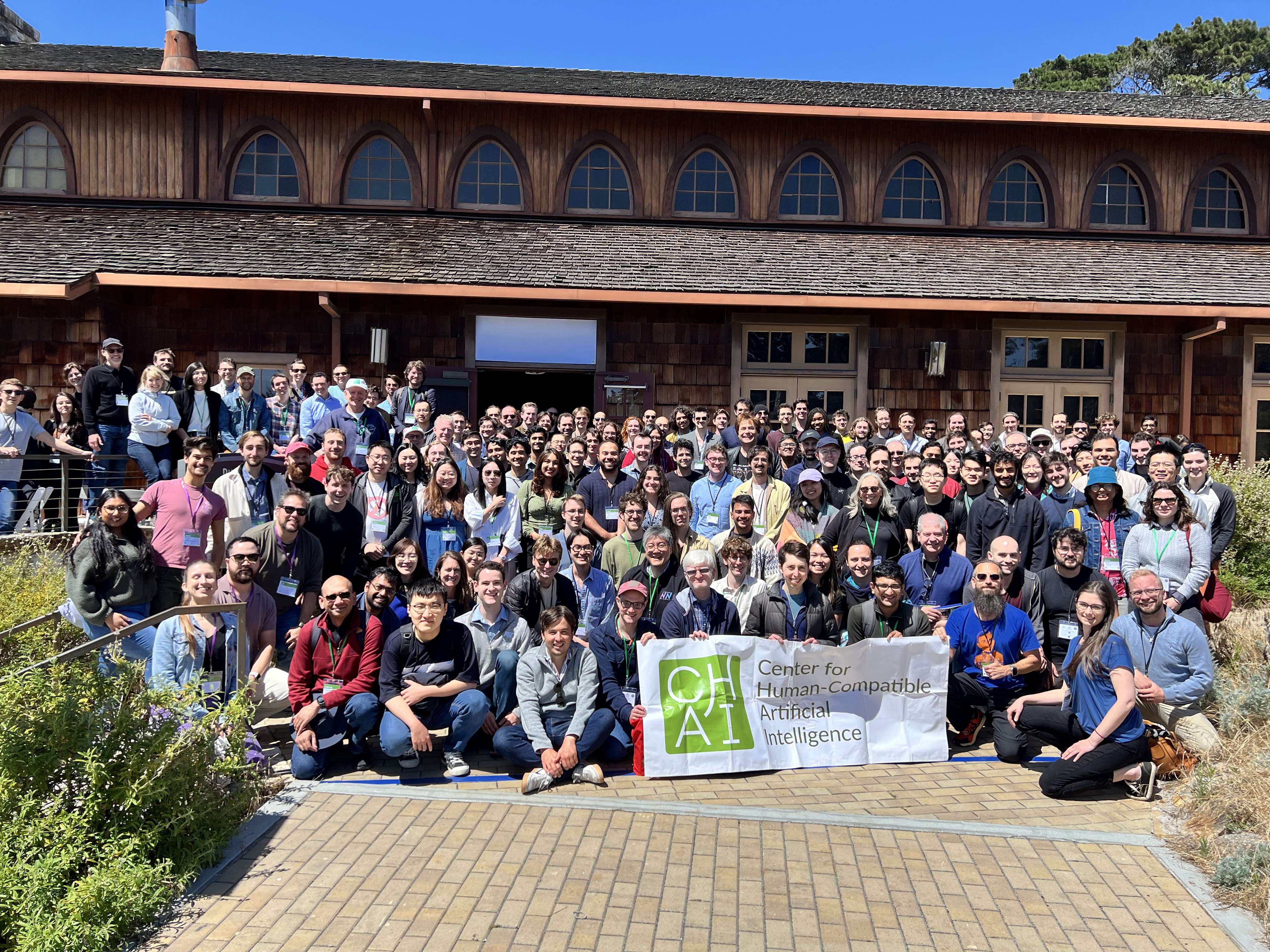
8th Annual CHAI Workshop
18 Jun 2024
CHAI held its 8th annual workshop at Asilomar Conference Grounds from June 13th to June 16th in Pacific Grove. The workshop had over 200 attendees which was the highest attendance to date. The workshop featured over 60 speakers and panelists and covered a wide array of topics from Societal Effects of AI to Adversarial Robustness.
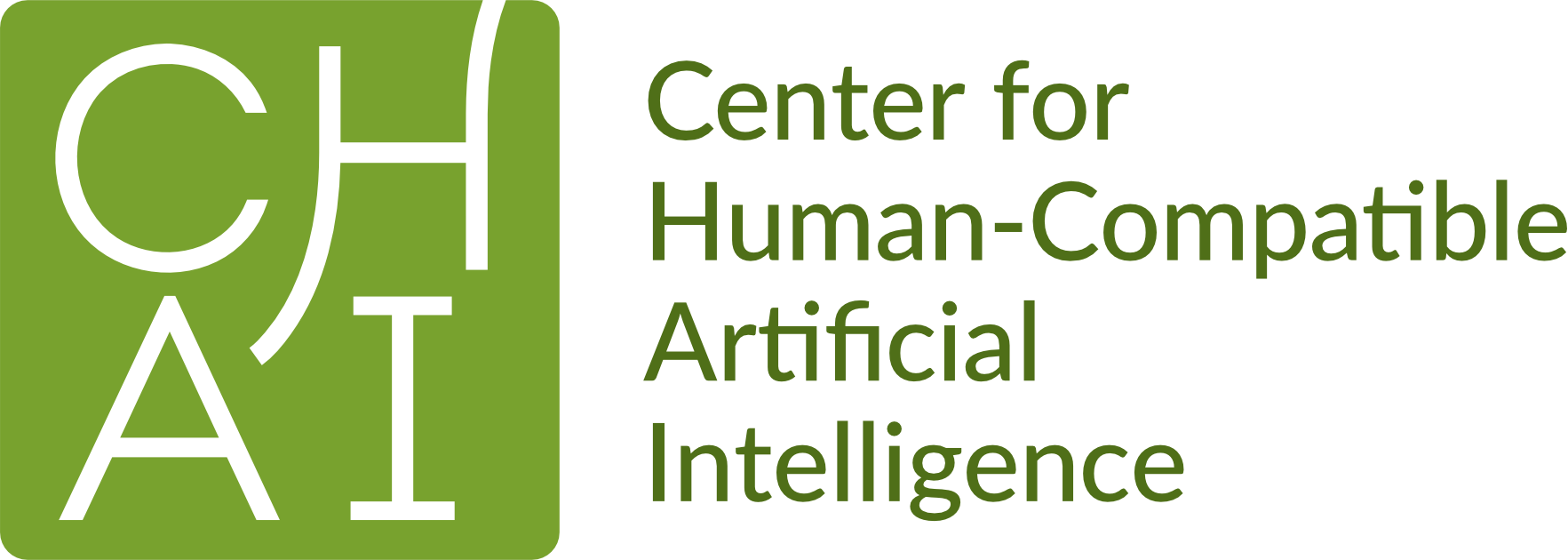
When Code Isn’t Law: Rethinking Regulation for Artificial Intelligence
05 Jun 2024
Brian Judge, Mark Nitzberg, and Stuart Russell wrote an article that was featured in Oxford Academic’s Policy and Society.
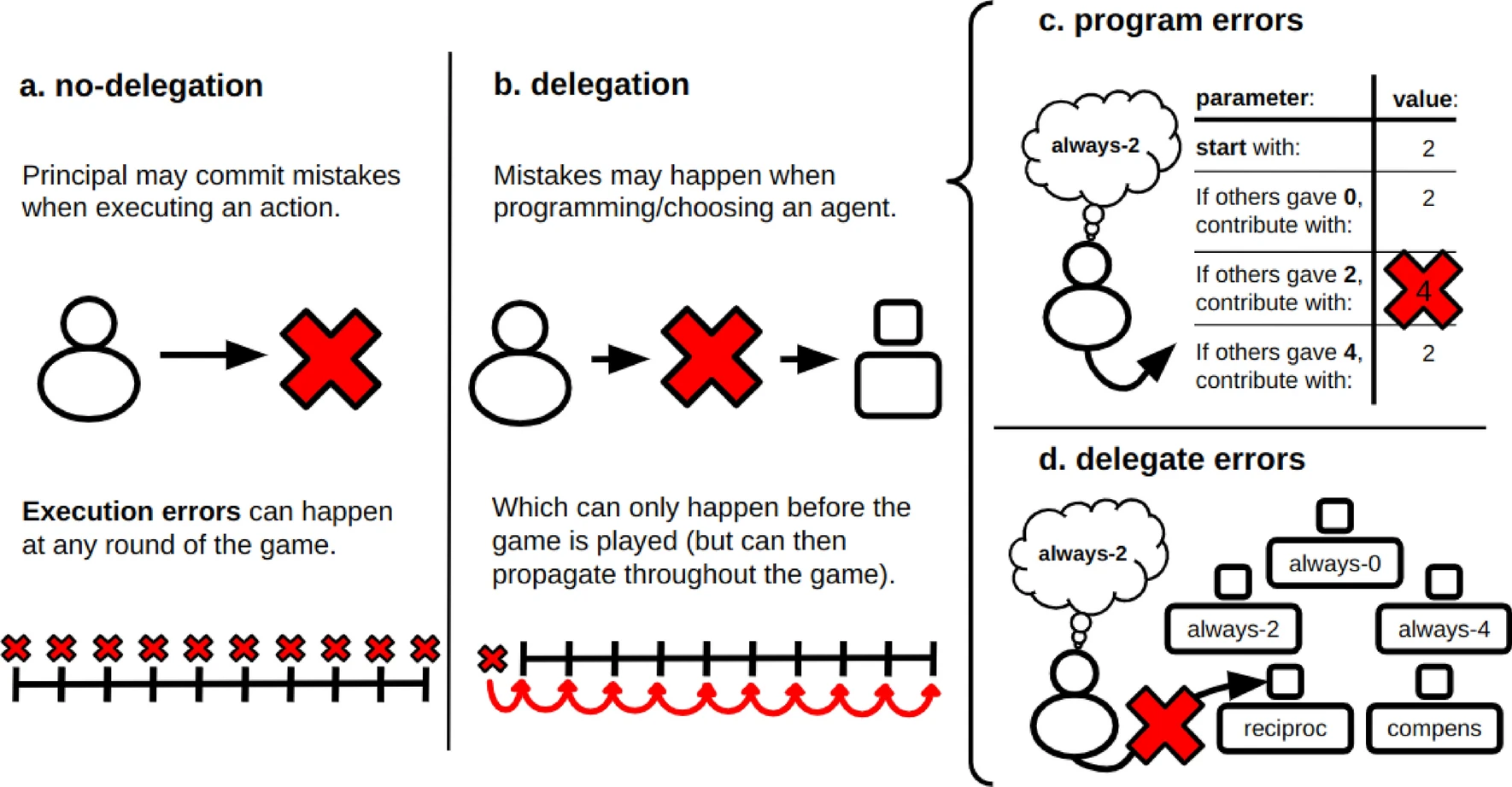
Committing to the wrong artificial delegate in a collective-risk dilemma is better than directly committing mistakes
13 May 2024
New research from computer scientists Inês Terrucha, Elias Fernández Domingos, Pieter Simoens, and Tom Lenaerts at the Vrije Universiteit Brussel, Université Libre de Bruxelles, and UC Berkeley’s Center for Human-Compatible AI

Reinforcement Learning with Human Feedback and Active Teacher Selection (RLHF and ATS)
30 Apr 2024
CHAI PhD graduate student, Rachel Freedman gave a presentation at Stanford University on critical new developments in AI safety, focusing on problems and potential solutions with Reinforcement Learning from Human Feedback (RLHF).