Even Superhuman Go AIs Have Surprising Failures Modes
28 Jul 2023
In March 2016, AlphaGo defeated the Go world champion Lee Sedol, winning four games to one. Machines had finally become superhuman at Go. Since then, Go-playing AI has only grown stronger. The supremacy of AI over humans seemed assured, with Lee Sedol commenting they are an “entity that cannot be defeated”. But in 2022, amateur Go player Kellin Pelrine defeated KataGo, a Go program that is even stronger than AlphaGo. How?
It turns out that even superhuman AIs have blind spots and can be tripped up by surprisingly simple tricks. In our new paper, we developed a way to automatically find vulnerabilities in a “victim” AI system by training an adversary AI system to beat the victim. With this approach, we found that KataGo systematically misevaluates large cyclically connected groups of stones. We also found that other superhuman Go bots including ELF OpenGo, Leela Zero and Fine Art suffer from a similar blindspot. Although such positions rarely occur in human games, they can be reliably created by executing a straightforward strategy. Indeed, the strategy is simple enough that you can teach it to a human who can then defeat these Go bots unaided.
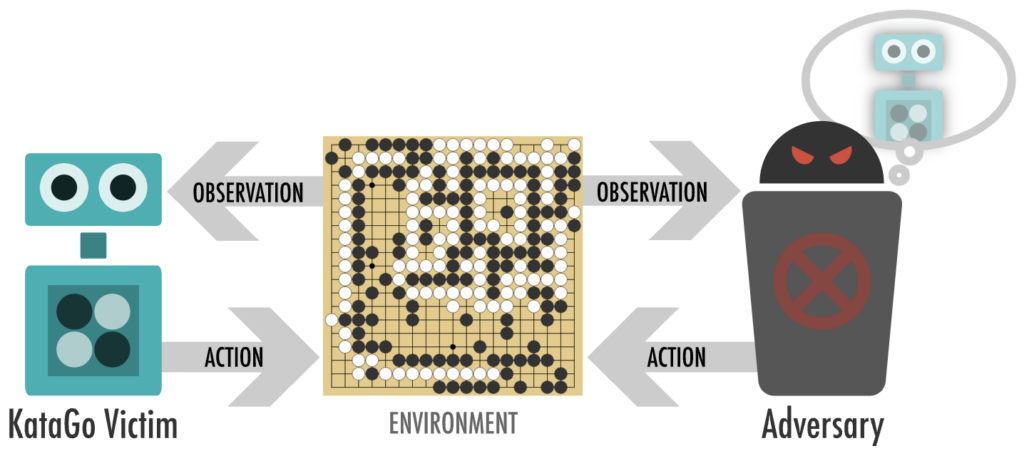
The victim and adversary take turns playing a game of Go. The adversary is able to sample moves the victim is likely to take, but otherwise has no special powers, and can only play legal Go moves.
Our AI system (that we call the adversary) can beat a superhuman version of KataGo in 94 out of 100 games, despite requiring only 8% of the computational power used to train that version of KataGo. We found two separate exploits: one where the adversary tricks KataGo into passing prematurely, and another that involves coaxing KataGo into confidently building an unsafe circular group that can be captured. Go enthusiasts can read an analysis of these games on the project website.
Our results also give some general lessons about AI outside of Go. Many AI systems, from image classifiers to natural language processing systems, are vulnerable to adversarial inputs: seemingly innocuous changes such as adding imperceptible static to an image or a distractor sentence to a paragraph can crater the performance of AI systems while not affecting humans. Some have assumed that these vulnerabilities will go away when AI systems get capable enough—and that superhuman AIs will always be wise to such attacks. We’ve shown that this isn’t necessarily the case: systems can simultaneously surpass top human professionals in the common case while faring worse than a human amateur in certain situations.
This is concerning: if superhuman Go AIs can be hacked in this way, who’s to say that transformative AI systems of the future won’t also have vulnerabilities? This is clearly problematic when AI systems are deployed in high-stakes situations (like running critical infrastructure, or performing automated trades) where bad actors are incentivized to exploit them. More subtly, it also poses significant problems when an AI system is tasked with overseeing another AI system, such as a learned reward model being used to train a reinforcement learning policy, as the lack of robustness may cause the policy to capably pursue the wrong objective (so-called reward hacking).
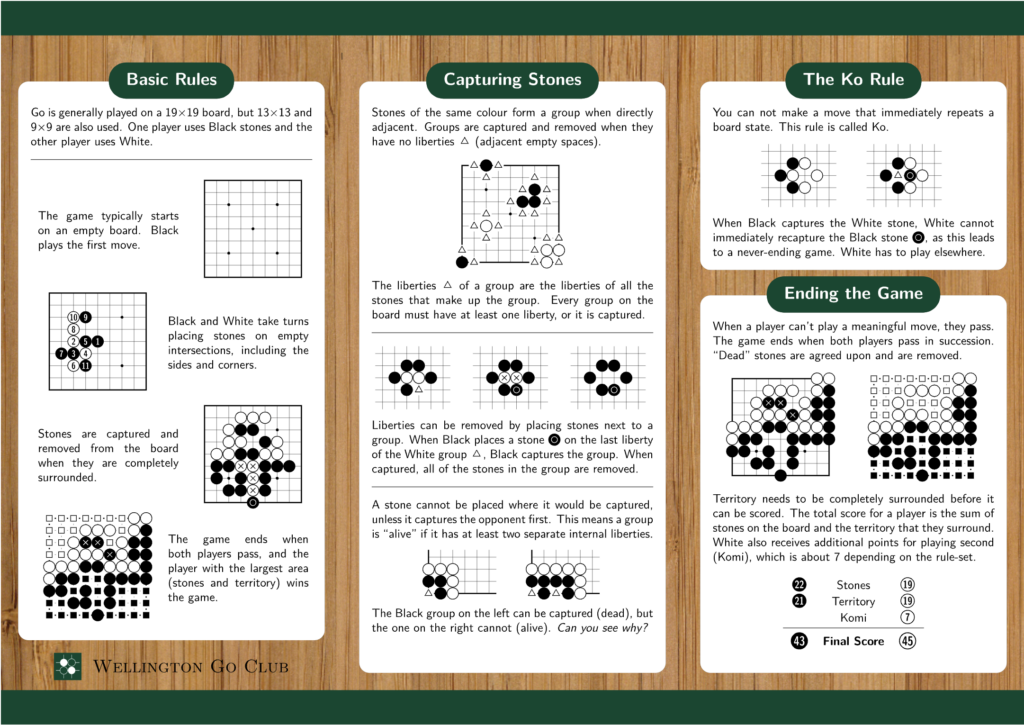
How to Find Vulnerabilities in Superhuman Go Bots
To design an attack we first need a threat model: assumptions about what information and resources the attacker (us) has access to. We assume we have access to the input/output behavior of KataGo, but not access to its inner workings (i.e. its weights). Specifically, we can show KataGo a board state (the position of all the stones on the board) and receive a (possibly stochastic) move that it would take in that position. This assumption is conservative: we can sample moves in this way from any publicly available Go program.
We focus on exploiting KataGo since, at the time of writing, it is the most capable publicly available Go program. Our approach is to train an adversary AI to find vulnerabilities in KataGo. We train the adversary in a similar way to how most modern Go bots are trained, via AlphaZero-style training (the section below gives a quick summary of this approach).
AlphaZero-style training
When you’re playing a game like Go or chess, there are, roughly speaking, two components to your decision making: intuition and simulation. On each turn, you’ll have some intuition of what kinds of moves would be good to play. For each promising move you consider, you’ll probably do a little simulation in your head of what is likely to unfold if you were to play that move. You’ll try to get into your opponent’s head and imagine what they’ll do in response, then what you would do next, and so on. If it’s an especially important move, you might simulate many different possible directions the game could go down.
AlphaZero and its successors are also roughly made of two parts corresponding to intuition and simulation. Intuition is achieved with a policy network: a neural network that takes in board states and outputs a probability distribution over possibly good next moves. Simulation is achieved with Monte Carlo Tree Search (MCTS), an algorithm that runs many simulations of the future of the game to find the move that is most likely to lead to a win.
On each turn, an AlphaZero agent generates some promising moves using the policy network, and then uses MCTS to simulate how each move would play out. Since it is not practical for MCTS to exhaustively evaluate every possible sequence of play, the policy network is used to steer MCTS in the direction of better moves. Additionally, a value network is used to heuristically evaluate board states so that MCTS does not need to simulate all the way to the end of the game. Typically, the policy and value networks are two heads of the same network, sharing weights at earlier layers.
The policy network is trained to match as closely as possible the distribution of moves output by MCTS, and the value network is trained to predict the outcome of games played by the agent. As the networks improve, so does MCTS; and with a stronger MCTS, the policy and value networks get a better source of signal to try and match. AlphaZero relies on this positive feedback loop between the policy network, value network, and MCTS.
Finally, the training data for AlphaZero-style agents is generated using self-play, where an agent plays many games against a copy of itself. Self-play works well because it creates a curriculum. A curriculum in machine learning is a sequence of gradually harder challenges for an agent to learn. When humans learn a skill like Go, they also need a gradual increase in difficulty to avoid getting stuck. Even the best Go players in the world had to start somewhere: If they only had other world champions to play against from the start, they would never have gotten where they are today. In self-play, the two players are always at the same level, so you get a curriculum naturally.
We modify the AlphaZero training procedure in a handful of ways. We want the adversary to be good at finding and exploiting bugs in KataGo, rather than learning generally good Go moves. So instead of playing against a copy of itself (so-called self-play), we pit the adversary against a static version of KataGo (which we dub victim-play).
We also modify the Monte-Carlo Tree Search (MCTS) procedure, illustrated below. In regular MCTS, moves are sampled from a single policy network. This works well in self-play, where both players are the same agent. But with victim-play, the adversary is playing against a potentially very different victim agent. We solve this by sampling from KataGo’s move distribution when it’s KataGo’s turn, and our policy network when it’s our turn.
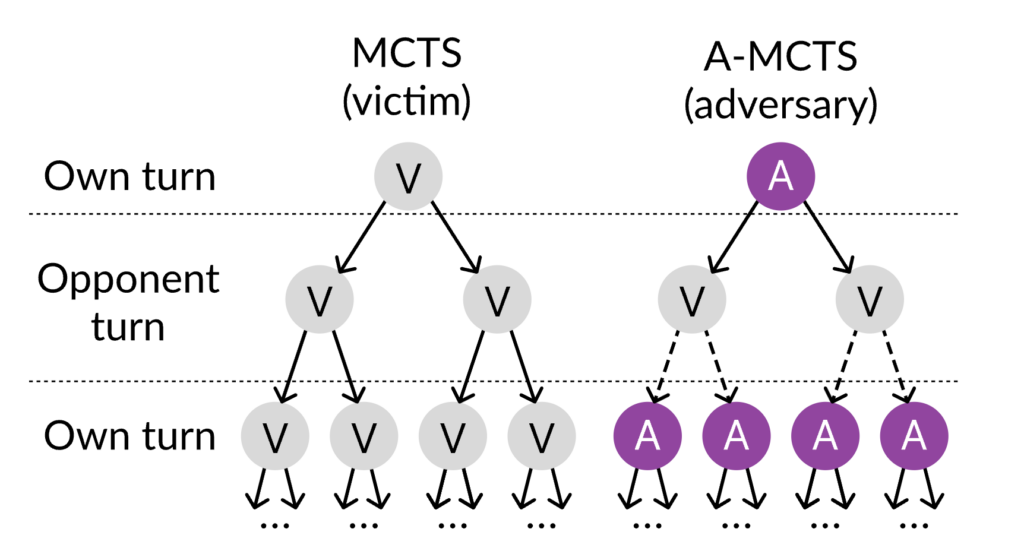
We also create a curriculum for the adversary by pitting it against a series of gradually more capable versions of KataGo. Whenever the adversary finds a way to consistently beat a KataGo version, we swap that version out for a better one. There are two ways to vary the skill of KataGo. Firstly, we use old versions (“checkpoints”) of KataGo’s neural network from various points of its training. Secondly, we vary the amount of search KataGo has: how many moves can be simulated during MCTS. The more moves that are simulated, the stronger KataGo is.
Our adversary relatively quickly learns to exploit KataGo playing without tree search (at the level of a top-100 European professional), achieving a greater than 95% win rate against KataGo after 200 million training steps (see orange line below). After this point, the curriculum continues to ramp up the difficulty every vertical dashed line. It takes another 300 million training steps to start reliably exploiting a strongly superhuman version of KataGo, playing with MCTS simultating 4096 moves for every move it makes (gray line). After this, the adversary learns to exploit successively harder victims with only small amounts of additional training data (although the computational requirements of generating the data successively increase as the victim’s search depth increases).
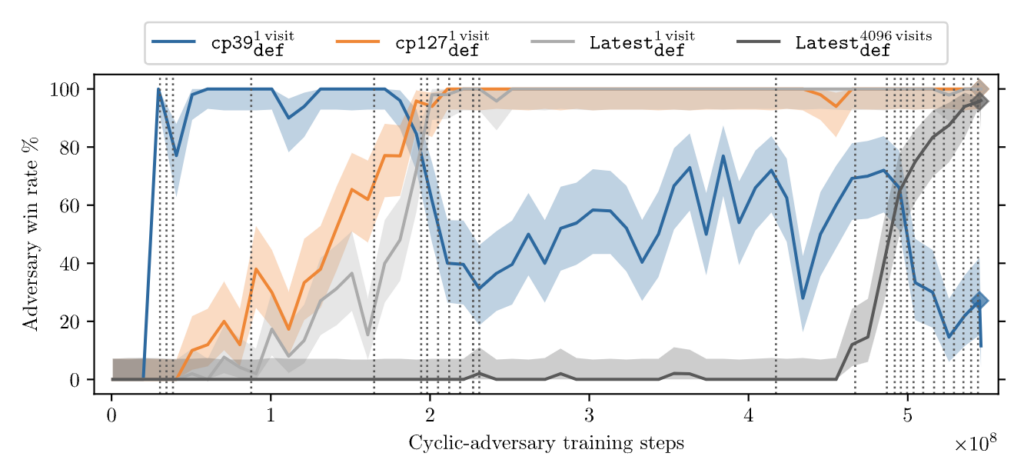
This adversarial training procedure discovered two distinct attacks that can reliably defeat KataGo: the pass attack and the cyclic attack. The pass attack works by tricking KataGo into passing, causing the game to end prematurely at a point favorable to the attacker. It is the less impressive of the two, as it can be patched with a hard-coded defense: expand the section below for more information on it. The cyclic attack on the other hand is a substantial vulnerability of both KataGo and other superhuman Go bots, which has yet to be fixed despite attempts by both our team and the lead developer of KataGo, David Wu. It works by exploiting KataGo’s misevaluation of large, cyclically connected groups of stones.
The Pass Attack
The first attack we discovered was the pass attack. It was found by an adversary trained with less than 1% of the computational resources required to train KataGo.
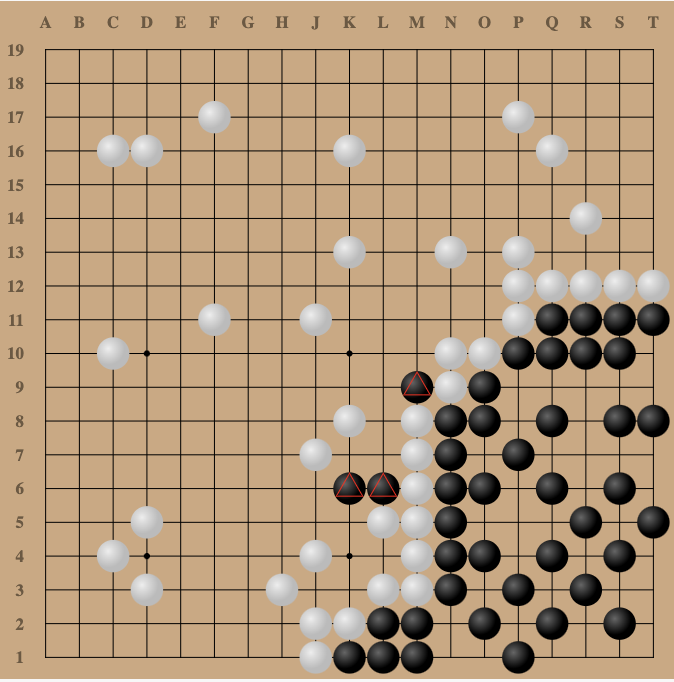
To perform the pass attack, the adversary focuses on securing a single corner as its territory, and lets KataGo spread across the rest of the board. Then the adversary plays some stones in KataGo’s territory to contest it (more on this later), and then passes its turn. KataGo then passes (since it seems to have much more territory than the adversary), ending the game. In Go, if both players pass one turn after the other, the game ends and the two players need to decide somehow which regions have been won by each player.
If this was a game between two humans, the players would decide based on what they expect would happen if they continue playing. In the above board state, if play continued, black would very likely secure the bottom right corner, and white would very likely secure the rest of the board, leading to white having much more territory than black. So the humans would agree that white (KataGo) has won.
But it’s different for games between AIs—we need to use some automated set of rules for deciding who has won at the end of a game. We chose to use KataGo’s version of Tromp-Taylor rules, which were the most frequently used ruleset during KataGo’s training. Under these rules, the game is scored as follows:
First, we remove stones that are guaranteed to be dead, as determined by Benson’s algorithm. Although a human would consider the three marked (△) black stones to be dead, they could live if white chose not to defend. So, the black stones are not removed from white’s territory.
Next, we mark every location on the board as belonging to black, white, or nobody. A location with a stone belongs to whichever color occupies that location. An empty region (formally a connected component of empty locations, connected along the cardinal directions) belongs to a color if that region only borders that single color. If an empty region borders both black and white stones, it is considered no-man’s land and belongs to neither player.
In the game above, all the empty locations in the lower-right belong to black. On the other hand, all of the remaining empty-space on the board is no-man’s land, since it borders both white stones and black’s marked black stones.
Finally, the total number of locations each player owns is counted, and whoever has more locations (modulo komi, extra points given to white to balance black making the first move) wins. In this case, black controls many more locations, so black wins.
When we published our results of this attack, we were met with skepticism from some members of the Go community as to whether this was a “real” exploit of KataGo, since it only affects play under computer rules. From a machine learning standpoint, this vulnerability is interesting regardless: KataGo has no inherent notion of how humans play Go, so the fact it is not vulnerable under human rules is largely a lucky coincidence. (Although the fact this vulnerability persisted for many years is perhaps a consequence of it not affecting human play. Had human players been able to win using this approach, it might have long ago been discovered and fixed.)
However, the attack is easily patched by hand-coding KataGo to not pass in unfavorable situations. We implemented this patch and then continued training our adversary against the patched KataGo. After another bout of training, we found a “true” adversarial attack on KataGo: the cyclic attack.
The Cyclic Attack
We identified the cyclic attack by training an adversary against a version of KataGo patched to avoid our first attack, the pass attack. The cyclic adversary first coaxes KataGo into building a group in a circular pattern. KataGo seems to think such groups are nearly indestructible, even though they are not. The cyclic adversary abuses this oversight to slowly re-surround KataGo’s cyclic group. KataGo only realizes the group is in danger when it is too late, and the adversary captures the group.
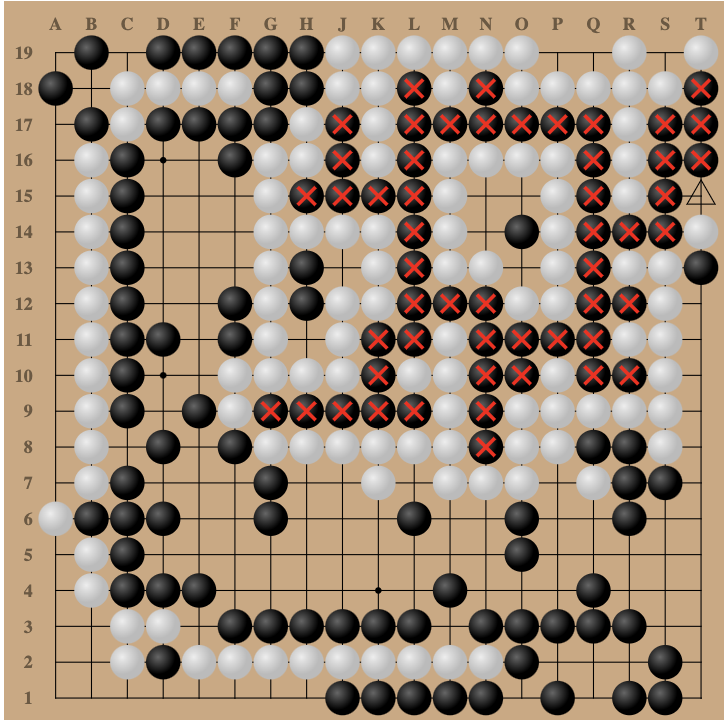
Using the cyclic attack, our adversary can reliably beat even strongly superhuman versions of KataGo. Let’s focus on three KataGo versions: one at the level of a top European professional (KataGo with no MCTS), one that is superhuman (KataGo with MCTS simulating 4096 moves for every move it makes), and one that is strongly superhuman (KataGo with MCTS simulating 10 million moves). Our adversary beat the human professional level bot in 100% of the games we ran, the superhuman bot 96% of the time, and the strongly superhuman bot 72% of the time. This is even though we trained our adversary with only 14% of the computational power used to train KataGo; moreover, our adversary only simulated 600 moves in all of these matches, far below the amount of search used by the superhuman and strongly superhuman versions of KataGo.
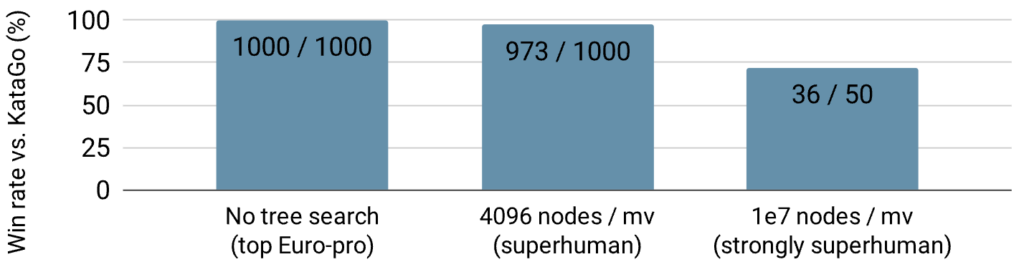
The win rate of our adversary against versions of KataGo with different amounts of search. KataGo versions become stronger going from left to right.
We were also interested in whether we could use this adversary, trained to beat KataGo, to defeat other superhuman Go-playing agents. We pitted this adversary against Leela Zero and ELF OpenGo without any training against these systems (a zero-shot transfer). The adversary beat Leela Zero 6% of the time and ELF OpenGo 4% of the time.
Although these win rates are modest, they demonstrate that other Go bots are vulnerable to the cyclic attack at least to some degree. Notably, these are superhuman AIs against which even the best human players in the world would struggle to win 1% of the time – so achieving a win rate of around 5% represents a significant vulnerability. This extends our original threat model: an attacker can conduct a black-box attack so long as they can obtain gray-box access to a sufficiently similar victim.
The cyclic attack is not just a specific set of moves that somehow exploit some arbitrary bug in KataGo; it’s a general and human-interpretable strategy. One of our authors Kellin, an amateur Go player, studied the behavior of our adversary to learn to play the cyclic attack himself. Kellin then used the cyclic attack to repeatedly beat superhuman versions of both KataGo and Leela Zero by himself. Many other Go enthusiasts have now used the cyclic attack to beat strong Go bots, including Sai (example) and Fine Art (example). You can learn the attack yourself with this video.
The Implications
The fact that the cyclic attack can be used to beat many different Go bots shows that the problem is not specific to KataGo. Moreover, in concurrent work, a team at DeepMind found a way to beat a human-expert level version of AlphaZero. The fact that two different teams could find two distinct exploits against distinct AI programs is strong evidence that the AlphaZero approach is intrinsically vulnerable. This in itself is interesting, but there are some more general lessons we can learn.
Adversarial attacks on neural networks have been known for nearly a decade, ever since researchers discovered that you can trick image classifiers by simply adding some imperceptible static to the image. Many have expected that these vulnerabilities in AI systems will disappear when the systems get suitably capable. Sure, an image classifier is tripped up by some static, but surely an image classifier that’s as capable as a human wouldn’t make such a dumb mistake?
Our results show that this is not necessarily the case. Just because a system is capable does not mean it is robust. Even superhuman AI systems can be tripped up by a human if the human knows its weaknesses. Another way to put this is that worst-case robustness (the ability to avoid negative outcomes in worst-case scenarios) is lagging behind average-case capabilities (the ability to do very well in the typical situation a system is trained in).
This has important implications for future deployment of AI systems. For now, it seems unwise to deploy AI systems in any security-critical setting, as even the most capable AI systems are vulnerable to a wide range of adversarial attack. Additionally, serious caution is required for any deployment in safety-critical settings: these failures highlight that even seemingly capable systems are often learning non-robust representations, which may cause the AI systems to fail in ways that are hard to anticipate due to inevitable discrepancies between their training and deployment environment.
These vulnerabilities also have important implications for AI alignment: the technical challenge of steering AI towards the goals of their user. Many proposed solutions to the alignment problem involve one “helper AI” providing a feedback signal steering the main AI system towards desirable behavior. Unfortunately, if the helper AI system is vulnerable to adversarial attack, then the main AI system will achieve a higher rating by the helper AI if it exploits the helper instead of achieving the desired task. To address this, we have proposed a new research program of fault-tolerant alignment strategies.
To summarize: we’ve found a way to systematically search for exploits against game-playing AI systems, and shown this approach can uncover surprisingly simple hacks that can reliably beat superhuman Go bots. All of the AlphaZero-style agents that we’ve studied are susceptible to the cyclic attack. There is a clear warning here about the powerful AI systems of the future: no matter how capable they seem, they may still fail in surprising ways. Adversarial testing and red teaming is essential for any high-stakes deployment, and finding new fault-tolerant approaches to AI may be necessary to avoid a chaotic future.
For more information, check out our ICML 2023 paper or the project website. If you are interested in working on problems related to adversarial robustness or AI safety more broadly, we’re hiring for research engineers and research scientists. We’d also be interested in exploring collaborations with researchers at other institutions: feel free to reach out to hello@far.ai.
Acknowledgements
Thanks to Lawrence Chan, Claudia Shi and Jean-Christophe Mourrat for feedback on earlier versions of this manuscript.